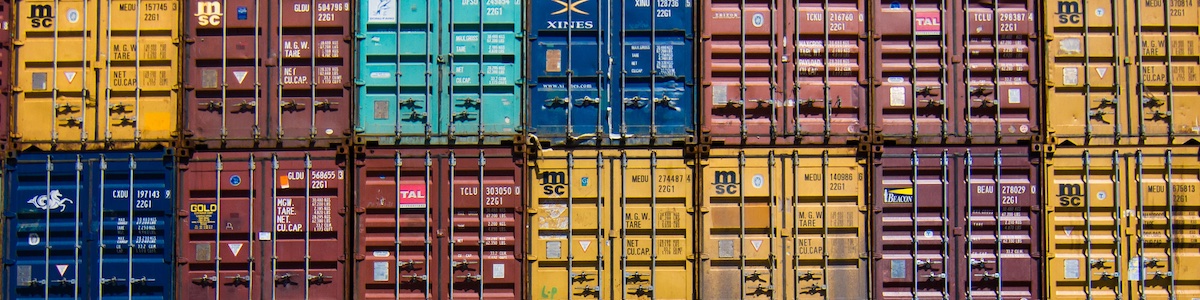
Eine Bestellung aufgeben
Projektprofil
Gesamte Kundenbedarfe und Bestellungen an Wechselgeldern auf Basis von Betriebsdaten für mehrere Monate für 5000 Locations prognostizieren. Im Rahmen der Supply Chain werden alle möglichen Bestellungen für die Bedarfe der Kunden vorhergesagt. Der Bestellprozess reduziert sich auf das Reporting für den Kunden damit und ermöglicht dispositive Freiheiten für den Logistiker
Auf einen Blick - Wesentliche Projektdaten
Dauer | Von 8/1/2019 to 11/29/2019 with about 4 months of full engagement | |
---|---|---|
Data and Tools | Market ? Logistics Sources • Bestelldatenbank • Kundeninformationen • Transportdatenbank (MS-SQL) von 8 Jahren • Wetter • GEO Informationen • Feiertagskalender • Bankfeiertage | |
Integration | •Web-API für Metadatenanreicherungen mit modellerzeugten Daten • Befüllung des Bestell-Shops mit den Predictions • Quality Reporting: Power BI • ML-Ops: Koppelung mit den Kundensystemen zur kontinuierlichen Übertragung von und zu unseren Modellen | |
AI Methoden | • Feature Engineering • Feature Importance • Deep Learning |
Hauptanwendungsfall
Kundenbedarfe/Bestellungen Wechselgeldern auf Basis von Betriebsdaten für mehrere Monate für 5000 Locations prognostizieren
Kundenmotivation / Lösungsansätze
Kundenbindung erhöhen
Automatisierungsgrad beim OneStop-Shop
Integrierte Planung von Bestellungen erhöhen
Forcastingklarheit für Kundengespräche/Budgetingsessions
Know-your-customer
AI-Ansatz
Eingesetzte AI Schlüsseltechnologien | Aufarbeitung (Erfassung, Bereinigung, Entflechtung) der Rohdaten und der komplexen Datenstruktur. Sehr gute Korrelation der zyklischen Komponenten und der Lieferfrequenz. | |
---|---|---|
Lösungsansatz | 3-layer Deep Learning Network mit zyklischen Features Kategorischen Embeddings | |
Projekt-Ansatz | Simply agile | |
Projekt-Typ | Operations/ML Ops | |
ML Integration und ML Betrieb | • Operation-Integration API • VIsualizationPower BI and Webshop Customer One-Stop Shop • ML-Ops at Customer Infrastructure onPremise |
Einblicke und Details
Exakte Vorhersage aus den Betriebsdaten mit dem Deep Learning Ansatz!
Genauigkeit des Ansatzes als Überprüfung der IST Bestellung (ggf. Ausreisser, Urlaub etc?)
Die Vorhersage erstreckt sich über ein ganzes Jahr. Damit wird Budgetierung für das kommende Jahr möglich
Fehlerbewertung ist essentiell für einen richtigen Ansatz. Feedback an den Kunden für die Ideale Paktierungsgröße wird mögich.
Erkennung einer Urlaubsabsicherung durch Bestellung ist erkennbar.