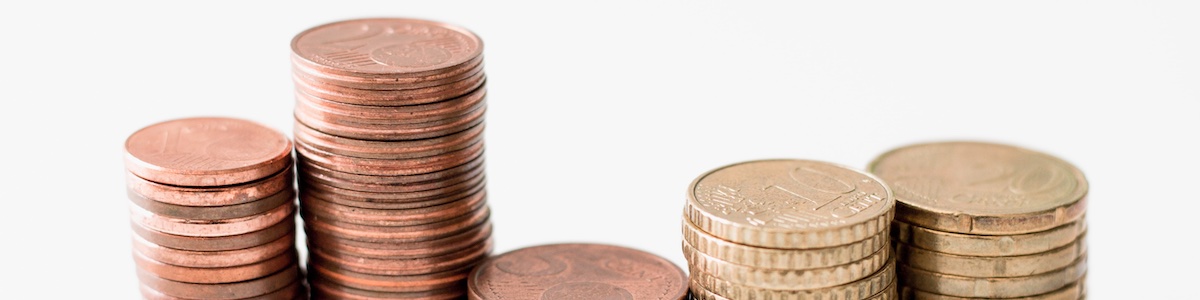
Getting the money in time - Logistics
Project Profile
At a glance - essential project data
Duration | From 11/1/2019 to 2/29/2020 with about 4 months of full engagement | |
---|---|---|
Data and Tools | Market - Logistics Source • Routing information • Transport database (MS-SQL) of 8 years • GEO information | |
Integration | • Web API for metadata enrichment with model generated data • Quality Reporting: Power BI • ML-Ops: Coupling with customer systems for continuous transfer to and from our models • Integration on the store floor as a benchmark for daily workload | |
AI Methods • Deep - Learning • Timeseries Models • Feature Engineering • Feature Importance • Unsupervised Training |
Engagement Use-Case
Packaging unit pickup orders with simultaneous forecasting of content quantity based on operational data for two weeks in advance for >8000 pickups predictable.
Client motivation / Solution aims
Optimization of approach frequencies and routes
Optimization of resources for counting routes
Efficiency for ShopFloor planning
AI Approach
AI key technology used in our solution | Great effort in obtaining domain knowledge (shift operation, closing/stop date, approach schedules, etc). Corona constrained optimization with 2-model strategy. | |
---|---|---|
Solution Approach | Decision Tree based ML Timeseries models (market/day based) | |
Project Approach | Simply agile | |
Project Type | Operations/ML Ops | |
ML Integration and ML Operations | • Operations Integration API • VIsualizationPowerBI and Shop-Floor Display • Capacity Management • ML Ops at Customer Infrastructure onPremise |
Insights and Details
Models can only be as good as the ACTUAL situation itself generates errors (false runs)
During COVID it was possible to deduce from the returns which locations had closed or will close. Here Analytics is a right choice for evaluation of the ACTUAL situation.
The prediction landed on the store floor. So the resource usage can be optimized by a prediction!